Accepted Paper at CVPR
- 01 February 2020
- Autonomous Learning
Our paper on optimizing rank-based metrics with blackbox differentiation received flawless reviews and got accepted at CVPR 2020
Abstract
Rank-based metrics are some of the most widely used criteria for performance evaluation of computervision models. Despite years of effort, direct optimization for these metrics remains a challengedue to their non-differentiable and non-decomposable nature. We present an efficient, theoreticallysound, and general method for differentiating rank-based metrics with mini-batch gradient descent.In addition, we address optimization instability and sparsity of the supervision signal that both arisefrom using rank-based metrics as optimization targets. Resulting losses based on recall and AveragePrecision are applied to image retrieval and object detection tasks. We obtain performance thatis competitive with state-of-the-art on standard image retrieval datasets and consistently improveperformance of near state-of-the-art object detectors.
arXiv version
People
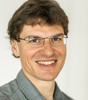